Published on 27 June 2023
An essential resource in danger
Date palms and Canary palms have been exploited since ancient time for the production of fruits and syrup, for their ornamental qualities and for many other uses (crop shading, basketry, fuel, construction, etc.) in the Mediterranean basin and the Middle East. Currently, they cover an estimated 1.3 million hectares. Unfortunately, these plants, not trees in the botanical sense despite their size, are under serious threat from a devastating pest. It's a beetle, the Red Palm Weevil (Rhynchophorus ferrugineus), which is spreading rapidly throughout the world, particularly via international trade of infested plants.
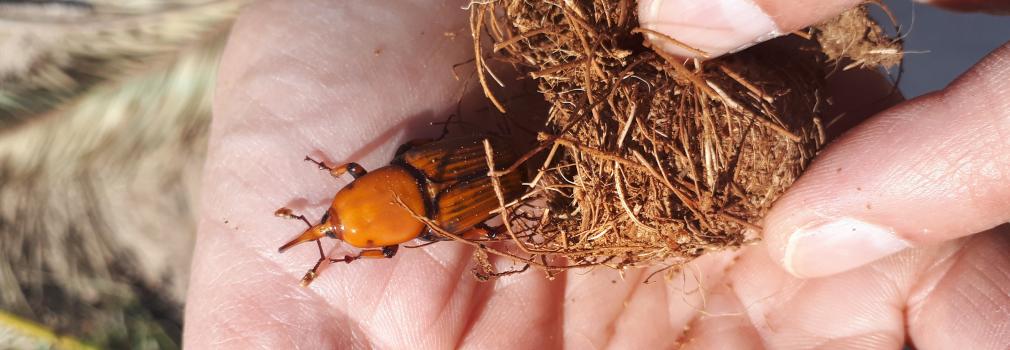
The red palm weevil is a fearsome pest of palm trees. The economic and environmental impacts of its attacks are considerable, with consequences for the food security and livelihoods of rural communities in date palm oases.
Early detection and remote sensing
Considering the importance of these palms for the socio-economic development of the regions mentioned, it is essential to define effective control strategies against this 'palm killer' in order to avoid a crisis situation. These strategies are based on monitoring and early detection of infestation, which are crucial to the survival of the palm trees. However, externally visible signs of weevil attack do not appear until long after infestation. This characteristic, combined with the wide spatial distribution of palms, argues in favor of using remote sensing as a tool to monitor palm health at a large scale. The STEREO III project PALMWATCH has therefore investigated wether airborne and space-based remote sensing techniques can be used to detect Red Weevil infestation in palms.
![]() |
![]() |
A palm detector learning by itself
Information on the number and distribution of infested palms is scarce. The team therefore began by training a palm detector based on deep learning to geolocate individual palm trees on aerial RGB (Red Green Blue, i.e. true color) images covering more than five thousand km2 in the Alicante province, an iconic palm-growing region.
The first step was to manually annotate the crowns of date and Canary palms in some of these images. This allowed the team to train the deep-learning object detector so that it can classify and locate palm crowns (and also delimit each tree) automatically . The detector is based on Convolutional Neural Networks (CNN), which have been promoted in the last few years in the remote sensing domain since they are designed to process data in the form of multiple networks (or arrays), as is the case with multi-band remote sensing data. Also, as CNN directly learns from imagery, the expert knowledge needed to extract features necessary in conventional machine learning methods is eliminated.
Results of the detection of Phoenix palms from an RGB orthophoto acquired in 2018 on the study site. The manual annotations are presented as circles; the magenta, blue, green, and orange boxes are the results obtained by different models. The average accuracy (AP) obtained by each model is shown in the corner of each image. The numbers inside the predictions correspond to the probability score that the object is indeed a palm tree. Only predictions with a score greater than 0.5 are shown.
© Institut Cartogràfic Valencià, Generalitat; OrtoPNOA 2018 CC BY 4.0 www.scne.es
Precise inventory on a large scale
In a second step, the researchers applied the detector over the complete imagery dataset of Alicante to finally obtain an inventory of each of the Phoenix palms (date palms and Canary palms) in the region.
Experimental results showed that the trained palm detection model provides a fast and straighforward method to geolocate isolated and densely planted palms from high-resolution RGB aerial imagery. Moreover, it allows conducting palm tree inventories at a wide scale and reduced cost compared to tree inventories using field-base measurements. The detector's performance showed a mapping capacity to geolocate palms in different scenes achieving 86% precision. In total, 1,505,605 palm trees were mapped over an area of 5,816 km2. The inventory produced as part of the project is freely accessible and can be consulted using the Palmwatch Earth Engine visualization software. It provides a baseline for the detection of pest-infested palms during more detailed inspections.
To this end, regular field and drone flights campaigns were carried out in an experimental palm tree plot to find the most appropriate method for drone-based detection of palms attacked by the red weevil. The results of these campaigns demonstrated that continuous monitoring the evolution of palm tree is more informative than monitoring healthy and infested palm trees at one single date. Furthermore, thermal drone imagery was shown to be more effective in early detection of disease symptoms compared to RGB or multispectral images.
Early detection of red weevil attacks remains a major challenge, but this study has paved the way for future research that will provide users with tools for early detection of the disease using remote sensing data.
More information
STEREO III project PALMWATCH (Red Palm Weevil infection detection with Remote Sensing)